We have passed the approval for our Horizon-application “ProcTwin”. Once we have completed the necessary documents and signatures, we will have the project entirely secured. Next few weeks will require some signing of documents for this.
The HiS budget is around EUR 592 000 over 4 years, and covers one PhD student and a good amount of work time for two senior researchers too. The total budget, with 12 partners is around EUR 4 826 000.
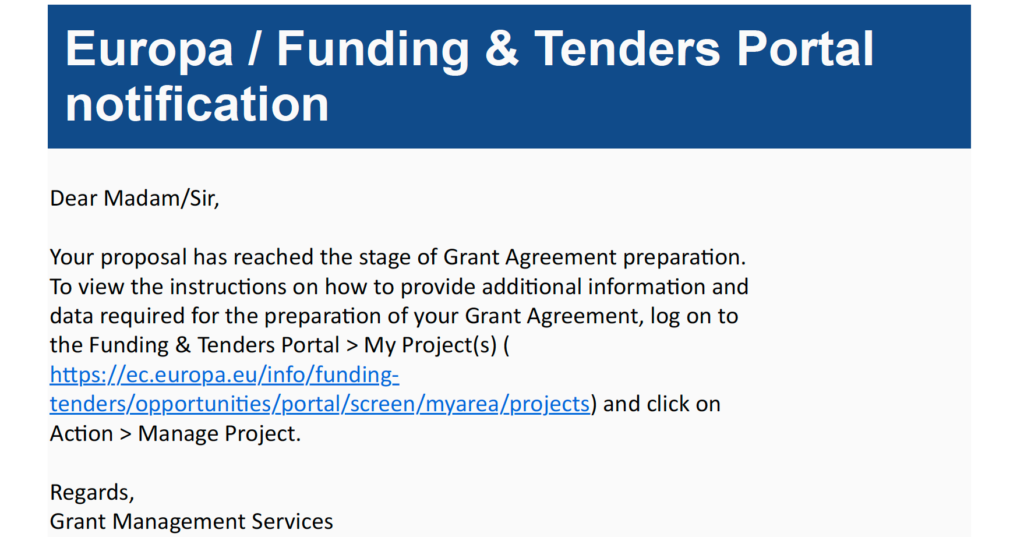
Project abstract:
ProcTwin aims to develop a demonstration platform to predict and visualize best use of multiple processing steps in a steel manufacturing chain. The methodology includes intelligent coupling of interconnected processing steps by numerical simulation, soft sensors, process data and distributed machine learning. Integrated numerical modelling that captures the interactions, relations, and feedback loops between various processing stations enables prediction for smart optimization of energy efficiency and product quality in the steel manufacturing. Continuous casting, reheating, hot metal working, quenching and leveling processes are examples that are controlled separately but strongly interconnected in terms of parameters. These processes serve as objective functions in two parallel use cases at Celsa (ES) and SSAB (SW). It is well known that process optimization can have a significant effect on reducing carbon footprint in steel production, and implementing new digital tools will enable a faster transition towards sustainable industry. ProcTwin is divided in clear work packages to reach the objectives: one is adaption of existing physically based numerical models of each process step to generate critical data that is impossible to measure or observe. Another is development of novel sensors and data integration for a secure and effective sharing industrial data. The innovative concept of ProcTwin is development of distributed machine learning to predict the process chains with large amounts of parameters. Lastly, these technologies will be combined through a demonstrator platform to model the manufacturing processes and enable control for increased product quality, energy efficiency and operator support.
The main idea is that instead of optimising each subsequent process component’s AI model separately, there is a benefit to have a distributed AI model that learns how to share information between such local AI models, to optimise the overall goal. We apply this in a chain of processes for a certain production output quality. The concept is meant to be used in steel manufacturing production lines, but the general case can be applied wherever there is a need for distributed AI with interacting and collaborating components that need to share knowledge for a common goal. The challenge is to learn what model knowledge to exchange, and how, to reach the common goal.